Understanding What is the Difference Between Business Intelligence and Data Science
- 12 December 2024
Table of contents
If you’re curious about what is the difference between business intelligence and data science, you’re not alone. Both fields harness data for decision-making, but they serve different purposes. Business Intelligence analyzes historical data to improve current operations, while Data Science predicts future trends using advanced algorithms. This article explores these distinctions in detail, helping you understand how each contributes to a data-driven strategy.
Key Takeaways
- Business Intelligence focuses on descriptive analysis of historical data to support decision-making, while Data Science employs advanced analytics to predict future trends and conditions.
The integration of Business Intelligence and Data Science enhances decision-making capabilities by combining real-time data insights with predictive modeling, allowing for a more comprehensive understanding of business operations.
Future advancements in AI, machine learning, and cloud computing are set to shape the evolution of both Business Intelligence and Data Science, improving the accuracy, efficiency, and accessibility of data analytics.
Defining Business Intelligence
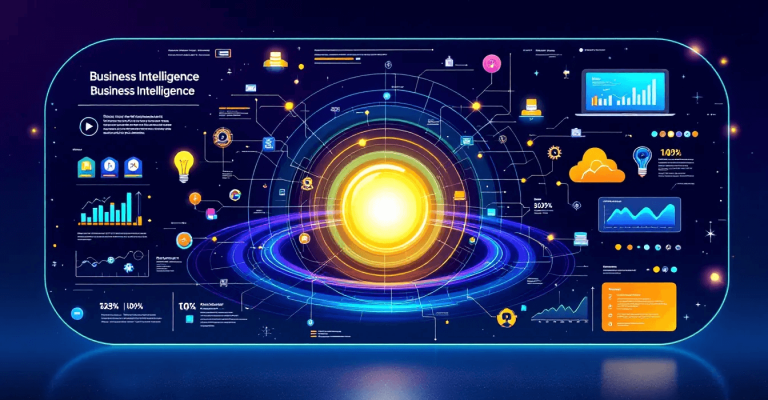
Business Intelligence (BI) is a set of technologies and processes designed to analyze business data and convert it into meaningful information for decision-making. The primary purpose of BI is to perform descriptive analysis, which means it focuses on historical data to help businesses understand past performance and make informed decisions based on facts rather than assumptions.
BI tools are crucial for creating reports and dashboards that present this data in an easily interpretable format. These tools support ad-hoc requests for actionable insights, enabling business users to make quick, data-driven decisions.
The advancements in BI technology, such as automation and data visualization, have significantly enhanced its capability to provide timely and accurate information. Accurate and timely data ensures the integrity and reliability of the insights generated.
Key Components of Business Intelligence
At the heart of Business Intelligence are several key components that work together to transform raw data into actionable insights. Data mining, which involves extracting valuable information from large datasets to identify patterns and correlations, is one key component. BI tools collect, govern, and transform data, preparing it for analysis and decision-making.
Predictive analytics uses historical data to forecast future trends and outcomes, helping businesses anticipate changes and make proactive decisions.
Data visualization tools allow users to create dashboards and reports, simplifying the interpretation of complex data and making it accessible to non-technical users. Modern BI tools with self-service capabilities empower business analysts and users to independently explore data analytics tools and derive insights.
Common Business Intelligence Tools
Several BI tools have become indispensable for enhancing data analysis and reporting. Tableau, known for its robust data visualization features, allows users to create interactive and shareable dashboards, making it a favorite among BI professionals.
Power BI, well-integrated with Microsoft products, offers a user-friendly interface for creating detailed reports and dashboards. These tools are pivotal in the data analysis process, enabling analysts to transform raw data into actionable insights.
Defining Data Science
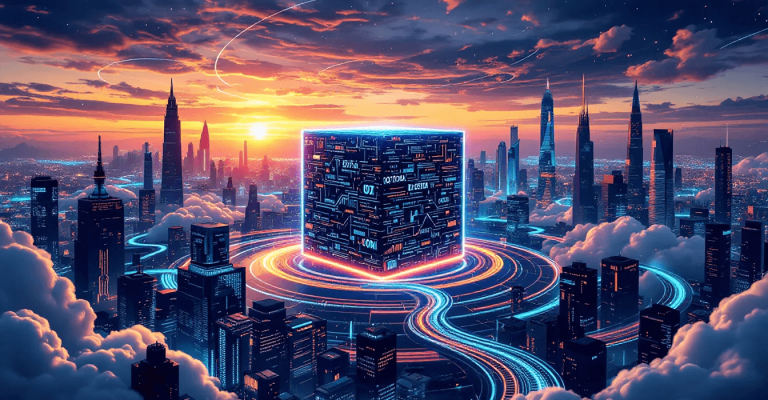
Data Science is a multidisciplinary field that uses scientific methods, algorithms, and processes to extract knowledge from structured and unstructured data. While BI focuses on descriptive analysis, Data Science aims to predict events or conditions based on hypotheses, leveraging advanced analytics and machine learning techniques to uncover patterns and forecasts.
Data Science spans long-term projects like building predictive models and forecasting outcomes, integrating disciplines such as mathematics, statistics, artificial intelligence, and computer engineering. This comprehensive approach enables deeper insights and more accurate predictions about future trends and events.
Core Elements of Data Science
Data Science relies on several core elements, including statistics, mathematics, and computer science, which collectively enable effective data analysis. Machine learning and artificial intelligence are critical technologies within Data Science, used to create predictive models and automate decision-making processes.
Data scientists commonly use programming languages such as Python, R, and SQL for manipulating and analyzing data. Predictive analytics in Data Science uses these tools to forecast future scenarios based on data, supporting strategic decision-making and planning.
Typical Data Science Applications
Data Science has a wide range of applications across various sectors, leveraging its predictive capabilities to enhance decision-making. In finance, it predicts market trends, assesses risks, and detects fraudulent activities. In healthcare, it predicts patient outcomes, personalizes treatment plans, and improves operational efficiencies.
Marketing and e-commerce companies use Data Science to analyze customer behavior, optimize pricing strategies, and predict sales trends. Uncovering hidden patterns in data enables organizations to make informed decisions that drive success and competitive advantage.
Comparing Business Intelligence and Data Science
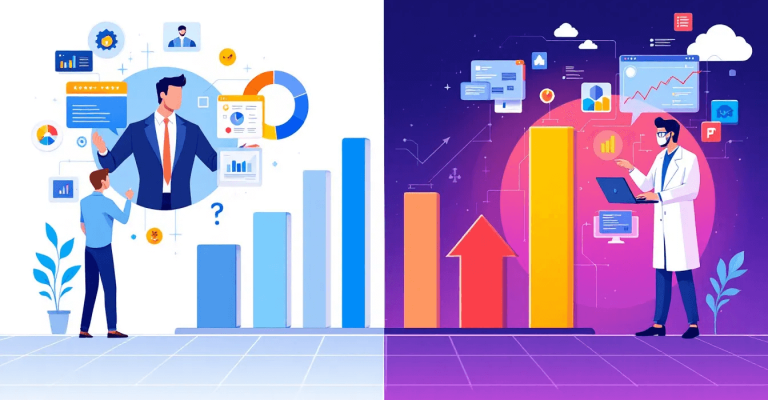
While both Business Intelligence and Data Science derive insights from data, they differ significantly in approach and outcome. Business Intelligence focuses on descriptive analysis, using historical data to inform operational decisions and create reports. In contrast, Data Science employs advanced algorithms and predictive models to forecast future trends and scenarios, offering a forward-looking perspective.
BI tools are designed for real-time operational decision-making, analyzing past and present data to provide actionable insights. Data Science integrates with BI to enhance decision-making by predicting future events and uncovering hidden patterns in data, addressing complex business challenges.
Analytical Focus
The analytical focus sets Business Intelligence and Data Science apart. BI primarily analyzes past events through descriptive analytics, generating reports that help organizations understand historical performance. This approach allows businesses to identify trends and make informed decisions based on past occurrences.
Data Science focuses on predicting future scenarios using advanced analytics and machine learning techniques. Predictive analytics in Data Science aims to forecast business trends, customer behaviors, and product success, providing a proactive approach to decision-making.
Integrating BI and Data Science provides organizations with a comprehensive view, combining historical insights with future predictions.
Data Types and Structures
Business Intelligence primarily focuses on structured data, which is well-organized and easily analyzed. This data is crucial for generating accurate reports and dashboards that inform business decisions based on past performance.
In contrast, Data Science deals with both structured and unstructured data, offering a broader scope for analysis. Data scientists utilize various data types, including semi-structured and unstructured data, to uncover deeper insights and develop predictive models.
Handling diverse data types enhances the predictive power of Data Science, making it a valuable complement to BI.
Skill Sets Required
The skill sets required for Business Intelligence and Data Science professionals differ significantly. BI professionals, such as business intelligence analysts, need business acumen and proficiency in self-service tools like Tableau to interpret data and create reports that support business decisions.
Data scientists require a robust technical skill set, including programming and statistical analysis. Their role as a data scientist involves developing complex algorithms and predictive models, requiring deep knowledge of mathematics and computer science.
Combining these diverse skill sets ensures that both BI and Data Science professionals effectively contribute to an organization’s data-driven decision-making processes.
Integration of Business Intelligence and Data Science
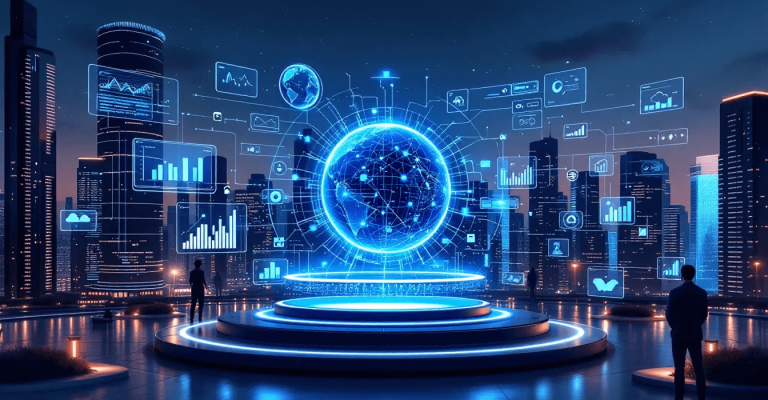
Integrating Business Intelligence with Data Science creates a powerful synergy that enhances organizational insights and decision-making. This integration allows organizations to tackle data complexity by combining structured and unstructured data for comprehensive analysis. BI supports data-driven decision-making with timely and accurate information, while Data Science enriches these insights by predicting future trends in data science business intelligence.
Successful integration requires executive commitment to ensure necessary resources and support for BI and Data Science initiatives. This combined approach helps organizations uncover hidden patterns and correlations, leading to better understanding and optimization of operations and customer behavior.
Benefits of Integration
Integrating Business Intelligence and Data Science offers numerous benefits that enhance organizational decision-making. One significant advantage is the empowerment of data-driven decisions, which reduces uncertainty and provides greater insights into business operations. Data governance, a critical component of BI, ensures that data quality and accessibility are maintained, further supporting informed decisions.
Additionally, integrating AI within BI enhances automated insights and predictive capabilities. Machine learning, a key aspect of Data Science, reveals insights that optimize business processes and improve decision-making. Merging BI with Data Science enables organizations to achieve a strategic advantage and improved performance.
Practical Examples of Integration
Practical examples of integrating Business Intelligence and Data Science highlight the tangible benefits. For instance, organizations can analyze past Request for Proposals (RFP) results to profile customers and projects, assisting in making informed decisions. This analysis helps in understanding which types of projects are more likely to succeed and why.
Another example involves using machine learning to predict the likelihood of winning future projects based on historical data. This predictive capability enhances organizational decision-making and forecasting, leading to better planning and resource allocation.
The synergy between BI and Data Science ultimately improves performance and provides a strategic advantage for organizations.
Future Trends in Business Intelligence and Data Science
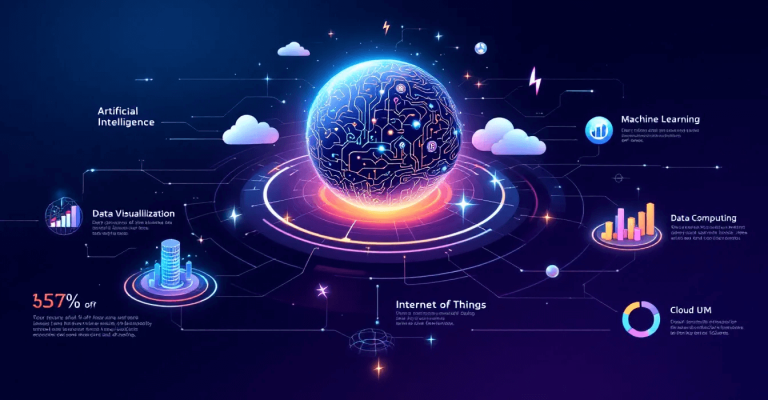
The future of Business Intelligence and Data Science is being shaped by advancements in AI, machine learning, and cloud computing. These technologies increasingly contribute to the accuracy and efficiency of BI and Data Science, allowing for better predictive analytics and automated insights. The role of AI and machine learning in enhancing prediction capabilities and streamlining workflows cannot be overstated.
Cloud computing facilitates the growth of BI and Data Science by providing scalable data storage and processing power. This enables organizations to leverage large datasets effectively, promoting collaboration and real-time access to business data. The ongoing evolution of these technologies promises to further enhance the capabilities and applications of BI and Data Science.
The Role of AI and Machine Learning
AI and machine learning are revolutionizing Business Intelligence and Data Science by enhancing prediction accuracy and streamlining workflows. Initiatives like Microsoft’s Project Brainwave make real-time AI calculations more feasible, significantly improving predictive analytics in BI. These technological advancements facilitate automated insights, enabling faster, data-driven decisions.
Future BI and Data Science in the cloud emphasize actionable insights over mere technological advancements. Integrating machine learning within BI tools helps uncover hidden patterns in data, improving decision-making processes and optimizing business operations.
The synergy between AI, machine learning, and BI tools is transforming how businesses utilize data to predict future trends and enhance strategic planning.
The Impact of Cloud Computing
Cloud computing plays a pivotal role in the evolution of Business Intelligence and Data Science. Cloud solutions allow for scalable data storage and processing, enabling organizations to handle growing data volume and complexity efficiently. This scalability is crucial for businesses needing to adapt quickly to changing data demands and leverage large datasets effectively.
Moreover, cloud-based BI solutions facilitate real-time access to business data, promoting seamless collaboration across departments and locations. This real-time capability enhances decision-making by providing up-to-date insights, ensuring business strategies are based on the most current information available.
As more organizations adopt cloud computing, the ability to manage and analyze data efficiently will continue to improve, driving better business outcomes.
Getting Started with BI and Data Science
Embarking on the journey of implementing Business Intelligence and Data Science requires a strategic approach. Successful data management begins with identifying essential data sources spread across various systems. This initial step is crucial for ensuring that all relevant data is considered during analysis, providing a comprehensive view of business operations.
Utilizing data effectively can drive businesses forward, turning data into a profitable asset. By setting clear objectives and selecting appropriate tools for data analysis and visualization, organizations can unlock the full potential of their data, leading to informed decisions and improved business outcomes.
Essential Features for Success
Several key features are essential for the successful implementation of BI and Data Science initiatives. The extract, transform, load (ETL) process is fundamental for effective data integration, ensuring that data from various sources is consolidated and ready for analysis. Additionally, the five main stages of data science—obtaining, scrubbing, exploring, modeling, and interpreting data—are crucial for maintaining high data quality and deriving meaningful insights.
Choosing the right visualization and reporting tools is vital for presenting data in a clear, actionable manner. Developing a structured BI framework helps in assessing requirements and creating a cohesive strategy, ensuring that all aspects of data management and analysis are aligned with business goals.
Steps to Implementation
Implementing BI and Data Science solutions involves several practical steps. First, it is essential to define clear objectives that guide the implementation process, ensuring that all efforts are aligned with the organization’s goals. Selecting appropriate tools for data analysis and visualization is critical, as these tools will determine the effectiveness of the insights derived.
An effective data integration method, such as Extract, Load, Transform (ELT), is necessary for managing large datasets efficiently. With the explosion of data quantity, cloud storage and processing solutions have become indispensable, providing the scalability needed to handle vast amounts of data.
By following these steps, organizations can successfully implement BI and Data Science solutions, leading to better data-driven decisions and improved business outcomes.
Summary
Understanding the differences between Business Intelligence and Data Science is crucial for leveraging their full potential. While BI focuses on descriptive analytics and past data to inform decisions, Data Science employs advanced algorithms and predictive models to forecast future trends. Integrating both fields provides a comprehensive approach to data analysis, enhancing decision-making and strategic planning.
The advancements in AI, machine learning, and cloud computing are shaping the future of BI and Data Science, offering more accurate predictions and scalable solutions. By implementing these technologies and following a structured approach, organizations can transform their data into actionable insights, driving better business outcomes and gaining a competitive edge.
Frequently Asked Questions
What is the primary focus of Business Intelligence?
Business Intelligence primarily focuses on descriptive analysis, leveraging historical data to guide operational decisions and generate reports effectively. Its aim is to transform data into actionable insights for better business outcomes.
How does Data Science differ from Business Intelligence?
Data Science employs advanced algorithms and predictive models to forecast future trends, while Business Intelligence concentrates on analyzing past data. This distinction highlights Data Science’s emphasis on proactive insights versus the reactive nature of Business Intelligence.
What are some common tools used in Business Intelligence?
Tableau and Power BI are commonly used tools in Business Intelligence for their strong data visualization and reporting capabilities. These tools facilitate informed decision-making through effective data analysis.
Why is the integration of BI and Data Science beneficial?
The integration of Business Intelligence (BI) and Data Science is beneficial as it merges historical data analysis with predictive analytics, thereby enhancing decision-making and delivering comprehensive insights. This synergy enables organizations to make informed decisions based on data-driven forecasts.
What future trends are shaping Business Intelligence and Data Science?
Advancements in AI and machine learning, along with the growing adoption of cloud computing for scalable data solutions, are key trends shaping the future of Business Intelligence and Data Science. These developments will enhance data processing capabilities and drive more informed decision-making.
Fun Fact!
Using Container Depot Management Software is like giving your shipping yard a brain! It tracks containers, optimizes space, and prevents costly errors, just like Tetris—but for real-world logistics! Without it, depots could quickly turn into a chaotic puzzle that’s impossible to solve!